
Back in the time, the bigger machines were believed to be faster. But it’s 2021, and the tables have turned. TinyML is an emerging trend that has completely dismissed the older technological patterns. In fact, according to global tech market advisory firm ABI Research, about 2.5 billion devices will be shipped with TinyML chipset by 2030.
The popularity of TinyML is reasonable. Not only has it made the computing at edge cheaper and more stable, but also has facilitated an improved response time, privacy, and low energy cost. This way TinyML has a great potential of transforming IoT devices completely. In this article, we will try to explain how this technology works. But first, let’s shed some light on what TinyML actually is. So without further ado, dive right in.
What is TinyML?
TinyML is short for Tiny machine learning. These are miniature ML algorithms that can be embedded in IoT devices. This software technology is mainly used to build low-power devices for running machine learning models.
Need for TinyML
TinyML has the potential to bring together extremely low-power systems and machine learning models. This will in return make way for more fantastic on-device ML. Today the world does not need colossal power-hungry devices. TinyML is the proof that good things do come in small packages.
How TinyML works?
Before the conversion stage, it is important to train the ML models for successful implementation. After this, comes the concept of TinyML. Let's take a look at how ML is converted to TinyML in the following four steps.
- Compression – After the training phase of the ML model, it can be compressed in a way that its accuracy does not change.
- Quantization – The above compressed model is then quantized so that it becomes compatible with the architecture of the device in which it is embedded further. Quantization handles any discrepancy between the chip and the embedded device.
- Encoding – This is an optional step because it does nothing but compresses the model further using Huffman encoding principles.
- Compilation – The final step is to compile the model into a format that can be interpreted and executed by neural network interpreters.
Challenges
As we know, all good things come at a certain cost. Similarly, there are a few challenges that will come your way while integrating TinyML into IoT devices. We have tried to highlight a few of them.
- The first and foremost hurdle you’ll face is to overcome all the technical challenges within edge computing. This can be quite tasking.
- Secondly, there are many differences between web-based and embedded technologies. These can be either in the context of deployment or execution.
- And thirdly, you will need a computational resource in order to get a precise and valid output.
TinyML and its applications across industries
As per Grandview Research, globally, more than 30 billion Microcontroller Units (MCUs) were shipped in 2019. This statistic has a clear link with the increasing popularity of embedded control systems and their applications in multifunctional devices such as vehicles, robots, medical devices, mobile radio, and different residential appliances. TinyML can have a huge impact on different industries. Take a look at how.
- Traffic Mobility: TinyML can help improve passenger safety as well as managing traffic effectively. The TinyML sensors placed strategically at different locations can be helpful in reducing jams and improving emergency response times.
- Manufacturing Industry: TinyML can be used in equipment monitoring. This way alerts can be sent out when preventive measures are necessary in the case of equipment failure.
- Agriculture Industry: TinyML can play a huge role in agriculture. This tech can be embedded in the wearables devices for the livestock. This way you can monitor vital parameters in time.
- Healthcare Industry: Just like in agriculture, the healthcare industry can also reap the benefits of TinyML. Apart from regularly monitoring the patients’ vitals like heart rate, body temperature, normal blood pressure, etc, in case of critical patients, it can be used to send out alerts in case of emergencies.
- Retail Industry: In the retail industry, TinyML can be a huge savior in case of Inventory management by monitoring shelves in stores and updating stock numbers automatically.
Conclusion
We hope that this blog gives you a certain clarity about TinyML as a technology of the future. We can see it slowly making a place among different technologies. Although it will take some time to take over the market completely, it has already taken over the IoT industry. And we can expect it to take over different markets soon.
Will TinyML be the next big thing on the technological front? Share your thoughts with us in the comment sections below.
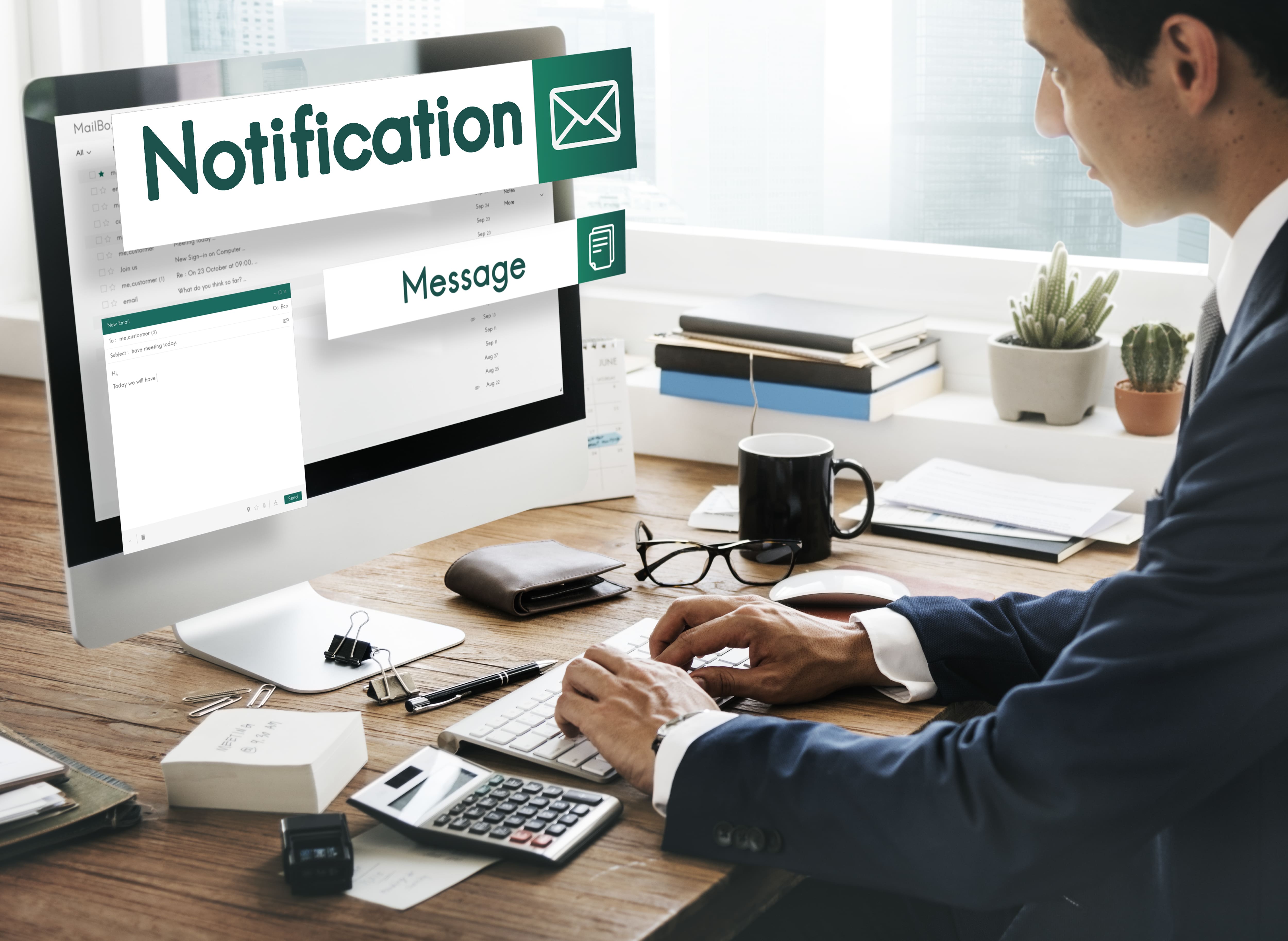