In 2024, the global big data analytics market in manufacturing was valued at approximately USD 9.07 billion and is projected to reach USD 19.25 billion by 2029, growing at a CAGR of 16.24%. However, the true value lies not just in adopting analytics technology but in orchestrating data as a strategic asset to drive operational resilience and sustained competitive advantage.
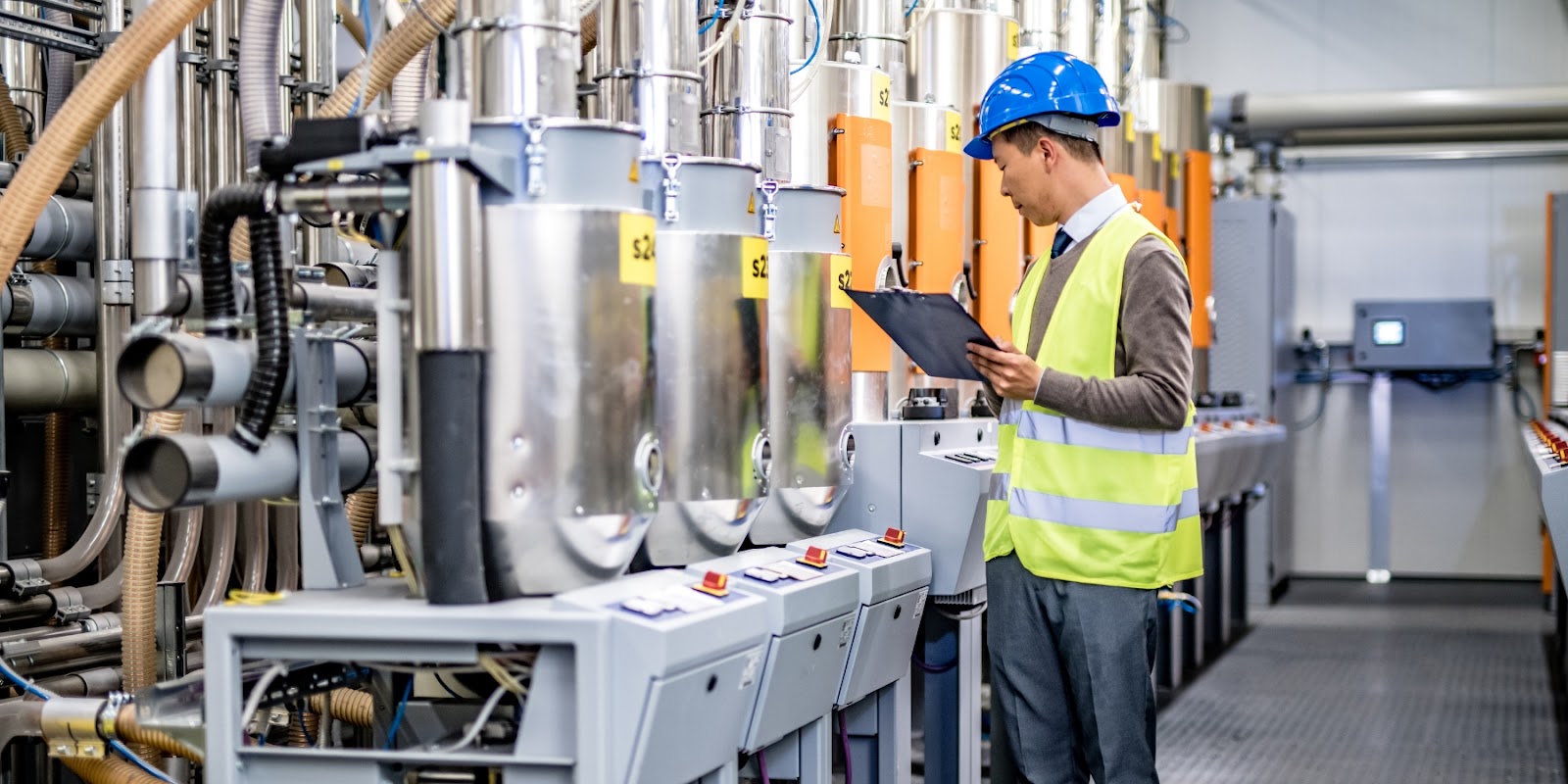
What is data analytics in manufacturing?
While data analytics is often described as “insights from data,” for manufacturing leaders it must be viewed through the lens of business outcomes: How does the data translate to measurable impact on cost, quality, agility, and innovation?
A mature data analytics capability integrates diverse datasets — machine telemetry, ERP, supply chain, quality, and customer feedback — to provide real-time decision intelligence. This enables leadership to move from reactive firefighting to proactive scenario planning, such as identifying supply chain vulnerabilities or simulating production adjustments under material shortages.
Effective analytics platforms also integrate with enterprise workflows and automation to embed decision support directly into daily operations — a capability that separates digital leaders from followers.
Why data analytics matters for the manufacturing industry
It is no longer sufficient to treat data analytics as a “nice-to-have” tech upgrade. Manufacturing leaders face critical industry shifts including:
- Supply chain disruptions: Analytics-driven visibility and predictive models reduce risk by flagging potential bottlenecks weeks in advance, rather than reacting to shortages after the fact.
- Rising input costs and margin pressure: Fine-grained cost-to-serve analytics enable precise identification of wasteful processes, unprofitable SKUs, or inefficient machine cycles to reclaim margin.
- Customer expectations for customization and speed: Data-driven demand sensing and flexible production planning allow faster, lower-risk responses to market changes.
- Workforce skills gaps and turnover: Embedding AI-powered decision tools alleviates dependency on scarce expert knowledge, enabling less experienced operators to maintain high performance.
For example, a leading automotive manufacturer reduced unplanned downtime by 20% and improved yield by 15% within 12 months after deploying a predictive analytics platform coupled with real-time operator alerts.
This strategic impact is why manufacturers investing in data analytics outperform peers—growing revenue at nearly double the rate and increasing EBITDA margins by 3-5 percentage points compared to industry averages.
Key use cases of data analytics in manufacturing
Data analytics is reshaping manufacturing by unlocking practical solutions that directly impact efficiency, cost, and quality. Understanding the most impactful use cases helps leaders prioritize investments and initiatives that deliver measurable returns.
1. Predictive maintenance to reduce downtime
Beyond reducing downtime, predictive maintenance programs deliver significant ROI by optimizing maintenance schedules to balance cost and risk.
For instance, a discrete manufacturer realized a 30% reduction in maintenance costs by shifting from time-based to condition-based maintenance driven by sensor analytics, while avoiding catastrophic failures that would have caused millions in lost revenue.
Business leaders must prioritize assets by criticality and financial exposure, then tailor analytics models accordingly — avoiding “big data for data’s sake” and focusing on risk-weighted asset management.
2. Process optimization through causal analysis
Surface-level metrics can be misleading. Advanced analytics that identify root causes of variability — such as correlation between environmental conditions, raw material lot variations, and output quality — enable precision interventions.
This drives continuous improvement cycles that materially reduce scrap rates and rework costs, with downstream benefits in customer satisfaction and warranty claims.
3. Demand-driven supply chain resilience
Manufacturers that embed real-time analytics on supplier risk signals (geopolitical events, logistics delays, price volatility) into procurement decisions are better positioned to mitigate supply shocks and cost spikes.
Strategic use of data here also enables dynamic inventory optimization that balances working capital against service levels, a critical lever for cash flow management in volatile markets.
4. Energy management as a competitive differentiator
Energy costs can represent 20-30% of total manufacturing expenses. Leveraging analytics to optimize machine scheduling for energy cost variability (time-of-use pricing, demand response) and identify wasteful consumption can improve margins significantly.
This also aligns with sustainability goals increasingly demanded by customers and regulators, creating market differentiation and reducing compliance risk.

Building a data-driven manufacturing environment
Business leaders must recognize that data infrastructure decisions have multi-year impacts on agility and cost. A poorly architected data platform can lead to siloed insights, slow query times, and prohibitive costs.
Adopting a modern cloud-based data lake architecture offers:
- Unified data access: Breaking down silos by centralizing all operational, transactional, and external data.
- Extensibility: Easily integrate new data sources, AI models, and third-party services.
- Cost transparency: Pay only for storage and compute needed, with scalable usage.
- Security: Enterprise-grade compliance and governance controls.
Effective partnerships with experienced providers in data lakes consulting ensure the platform supports both current and future analytics needs without costly rework.
Leveraging AI and generative AI in manufacturing
Beyond descriptive and predictive analytics, AI-driven prescriptive analytics and generative AI in manufacturing open new frontiers:
- Prescriptive models optimize complex tradeoffs in scheduling, quality, and supply chain in near real-time, enabling autonomous decision-making at scale.
- Generative AI assists R&D by rapidly designing novel components or simulating manufacturing process variants, dramatically shortening innovation cycles.
Manufacturers investing strategically in AI capabilities, guided by expert generative AI consulting, gain the first-mover advantage to disrupt markets and redefine operational excellence.
Best practices for manufacturing leaders to maximize analytics impact
- Align analytics strategy with top business priorities: Ensure initiatives directly support revenue growth, cost reduction, or risk mitigation.
- Drive cultural change: Empower teams with data literacy training and embed analytics into daily workflows.
- Measure outcomes rigorously: Track KPIs tied to analytics investments to guide continuous improvement.
- Adopt agile governance: Balance data security and compliance with innovation velocity.
- Partner with experts: Leverage external expertise in data analytics, AI, and cloud to accelerate transformation while managing risks.
Conclusion
For manufacturing business leaders, data analytics is a strategic imperative—not a technology experiment. It unlocks deeper operational insights, drives innovation, and safeguards competitiveness in an uncertain world.
Investing in scalable data infrastructure, AI capabilities, and organizational readiness enables manufacturers to:
- Predict and prevent costly disruptions
- Optimize resource utilization for improved margins
- Respond swiftly to evolving customer demands
- Foster innovation through AI-augmented R&D
At Applify, we help manufacturers architect future-proof data ecosystems, embed AI into operations, and leverage cloud agility for sustained growth. Get to touch with our experts with a free consultation to get started.